Artificial Intelligence and Human Rights
Government Use of AI
Background
Governments at all levels are using AI and Automated decision-making systems to expand or replace law enforcement functions, assist in public benefit decisions, and intake public complaints and comments. The use of these systems is poorly regulated and largely opaque.
Documents
Although the adoption of AI by federal government is growing—a February 2020 report found that nearly half of the 142 federal agencies studied had “experimented with AI and related machine learning tools”—many of the AI tools procured by government agencies have proven to be deeply flawed. The same goes for adoption of automated decision-making tools at the state and local levels. They’re used in law enforcement and the broader criminal legal cycle, in public benefit administration, in housing processes, and more. Certain states have pending legislation that would improve transparency and accountability of these tools state-wide, but none have passed yet.
AI and the Criminal Legal Cycle
In the criminal justice system, the deployment of AI and other algorithmic decision-making tools tends to exacerbate discriminatory policing patterns that already disadvantage minorities. A 2019 National Institute of Standards and Technology (“NIST”) study of facial recognition tools—which are typically “AI-based”—found that the systems were up to 100 times more likely to return a false positive for a non-white person than for a white person. Specifically, NIST found that “for one-to-many matching, the team saw higher rates of false positives for African American females,” a finding that is “particularly important because the consequences could include false accusations.” A separate study by Stanford University and MIT, which looked at three widely deployed commercial facial recognition tools, found an error rate of 34.7% for dark-skinned women compared to an error rate of 0.8% for light-skinned men. A review of Rekognition—an Amazon-owned facial recognition system marketed to law enforcement—revealed indications of racial bias and found that the system misidentified 28 members of U.S. Congress as convicted criminals. Similarly, AI and algorithmic decision-making tools used in pretrial dispositions, sentencing, and prison settings often yield inaccurate or biased results that perpetuate existing inequalities.
AI and Public Benefits
EPIC, through a freedom of information request, has obtained new records about the D.C. Department of Human Services’ use of automated systems to track and assign “risk score[s]” to recipients of public benefits. The documents show that DCDHS has contracted with Pondera, a Thomson Reuters subsidiary, for case management software and a tool known as “Fraudcaster.” Fraudcaster tracks location history and other information about people receiving public benefits, combining this information through “DHS data and pre-integrated third-party data sets” among other data sources to yield supposed risk scores. Factors that may cause the system to label someone as riskier include “travel[ing] long distances to retailers” and “display[ing] suspect activity.”
These documents are a concrete example of different tools used by government entities to help determine both eligibility for public benefits and to inform enforcement resources.
AI and Housing
In housing, there are several automated decision-making systems used: facial recognition and other biometric collection and analysis; algorithms deciding if someone is financially able to pay a mortgage or rent; profiles collected from opaque sources and combined into “trustworthiness” reports, and other instances of scoring and screening.
For lending, a rule that was struck down in 2020 created a defense to a discrimination claim under the Fair Housing Act where the “predictive analysis” tools used for lending decisions were not “overly restrictive on a protected class” or where they “accurately assessed risk.” The Judge explained that this regulation would “run the risk of effectively neutering disparate impact liability under the Fair Housing Act” in granting a preliminary injunction. Last October, EPIC and several others warned the federal housing agency that providing such a safe harbor for the use of algorithms in housing without imposing transparency, accountability, or data protection regulations would exacerbate harms to individuals subject to discrimination. The Alliance for Housing Justice called the rule “a vague, ambiguous exemption for predictive models that appears to confuse the concepts of disparate impact and intentional discrimination.”
For biometric identification, The No Biometric Barriers Housing Act was introduced in 2019 by Senator Booker (D-NJ), and Congresswomen Yvette D. Clarke (D-NY), Ayanna Pressley (D-MA) and Rashida Tlaib (D-MI) and would prohibit the usage of facial and biometric recognition in most federally funded public housing and direct the Department of Housing and Urban Development (HUD) to submit a report to Congress about the impact of the technology on it’s tenants.
For screening tools, they are used by landlords and housing authorities to help make decisions about whether to accept a tenant’s rent application. Companies who offer tenant screening tools collect, store, and select records for housing providers to use in evaluating tenants. These tenant screening reports often contain errors and misleading information, and there is little oversight of the companies’ record collection or matching practices. There are three major sources of error for tenant screening reports: lack of information in the records, record matching errors, and failure to update records databases. First, court records often lack enough information to accurately be matched to individuals. Second, screening reports continually match records to the wrong applicants. Companies may use overbroad search and matching practices, which lead to reports that contain records belonging to the wrong person. Frequently, these companies match records only based on first and last name, which can match a record to a different person. Third, these companies can collect or use outdated records, which do not accurately reflect updates to cases such as dismissals or sealing.
AI and Regulatory Enforcement Assistance
Many U.S. agencies such as the Securities Exchange Commission, Internal Revenue Service, and the Department of the Treasury use algorithms to help direct enforcement resources for potential fraud cases. These programs are detailed further in Government by Algorithm, a 2020 report from ACUS.
Recent Documents on Government Use of AI
-
Publications
Outsourced & Automated: How AI Companies Have Taken Over Government Decision-Making
Building on two years of state contracting research, EPIC publishes new report on the oft-forgotten world of government AI procurement.
Top Updates
Resources
-
Best Practices for Government Procurement of Data-Driven Technologies
Rashida Richardson | 2021
-
AI Procurement in a Box: AI Government Procurement Guidelines
World Economic Forum | 2020
-
Assembling Accountability: Algorithmic Impact Assessment for the Public Interest
Emanuel Moss et al. | 2021
-
The Algorithmic Auditing Trap
Mona Sloane | 2021
-
Algorithms and Economic Justice: A Taxonomy of Harms and a Path Forward for the Federal Trade Commission
Rebecca Kelly Slaughter et al. | 2021
-
The Right to Privacy in the Digital Age
United Nations High Comm’r for Human Rights | 2021
-
The False Comfort of Human Oversight as an Antidote to A.I. Harm
Ben Green & Amba Kak | 2021
-
Suspect Development Systems: Databasing Marginality and Enforcing Discipline
Amba Kak, Rashida Richardson | Forthcoming 2022
-
Racial Segregation and the Data-Driven Society: How Our Failure to Reckon with Root Causes Perpetuates Separate and Unequal Realities
Rashida Richardson | Forthcoming 2022
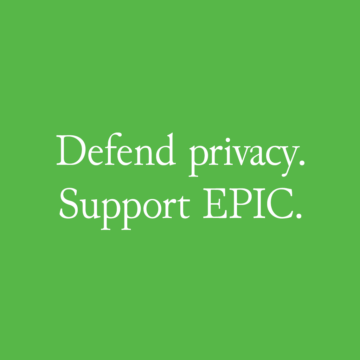
Support Our Work
EPIC's work is funded by the support of individuals like you, who help us to continue to protect privacy, open government, and democratic values in the information age.
Donate